Lithofacies Prediction – Rapid 3D Facies Modeling
Lithofacies Prediction – Rapid 3D Facies Modeling
Paradise Lithofacies Prediction Self-Guided Demo
Walk through a demo of the Paradise AI Lithofacies Prediction capabilities, which produces results in a fraction of the time compared to traditional methods.
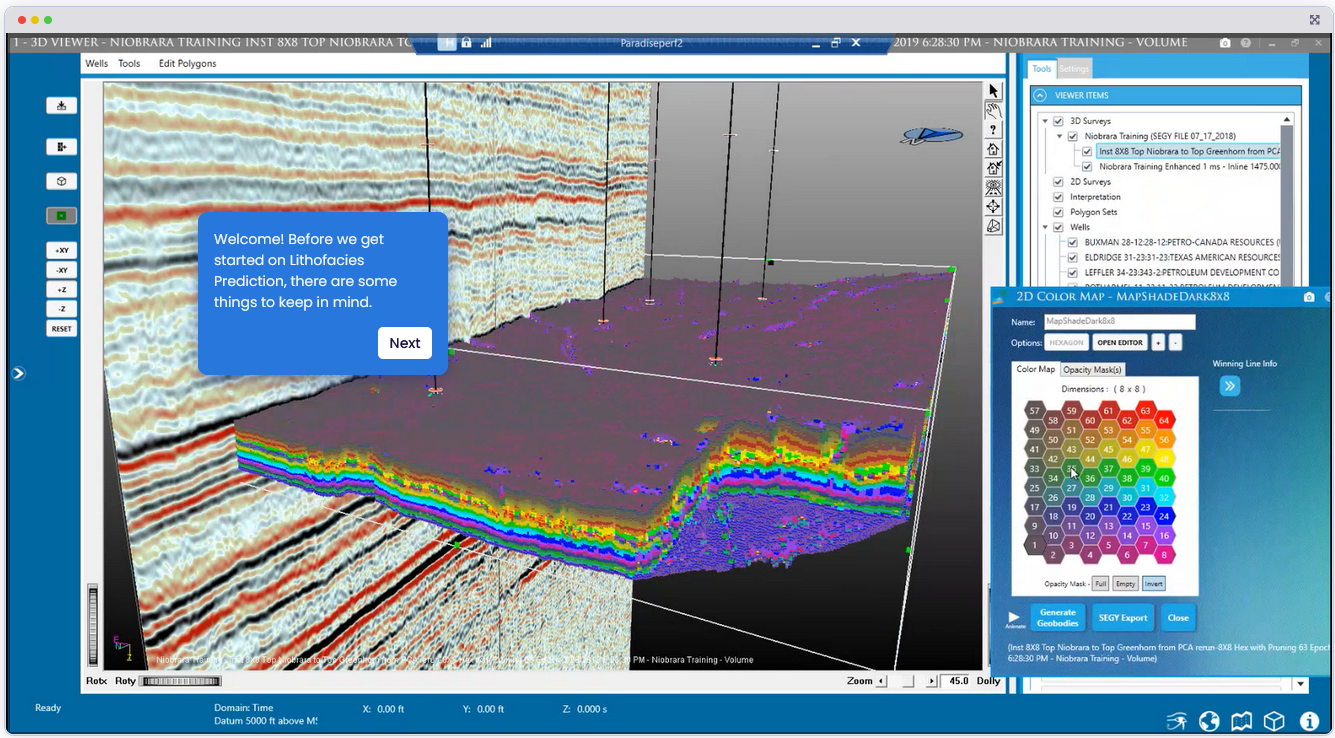
Lithofacies Prediction in Paradise delivers a breakthrough in reservoir modeling: it calculates the probability of designated lithologies at every location in the seismic volume, guided by well data. This workflow uses well log-derived lithofacies as training input and applies those classifications across the entire 3D seismic via the Paradise multi-attribute machine learning engine. The payoff is a high-resolution 3D lithofacies volume obtained in a fraction of the time of traditional inversion methods.
There is no need for building complex physical models or wavelets – the data-driven approach finds natural patterns relating seismic attributes to lithology, even in thin beds below conventional resolution limits. Geoscientists can thus predict facies distributions faster and at finer scale than ever before, dramatically reducing the cost and cycle time of reservoir characterization.
The lithofacies prediction results are easily visualized and analyzed in Paradise. By leveraging machine learning lithofacies prediction, teams gain a robust, quantitative facies model tied to real well observations – empowering more accurate geological interpretations across the asset.
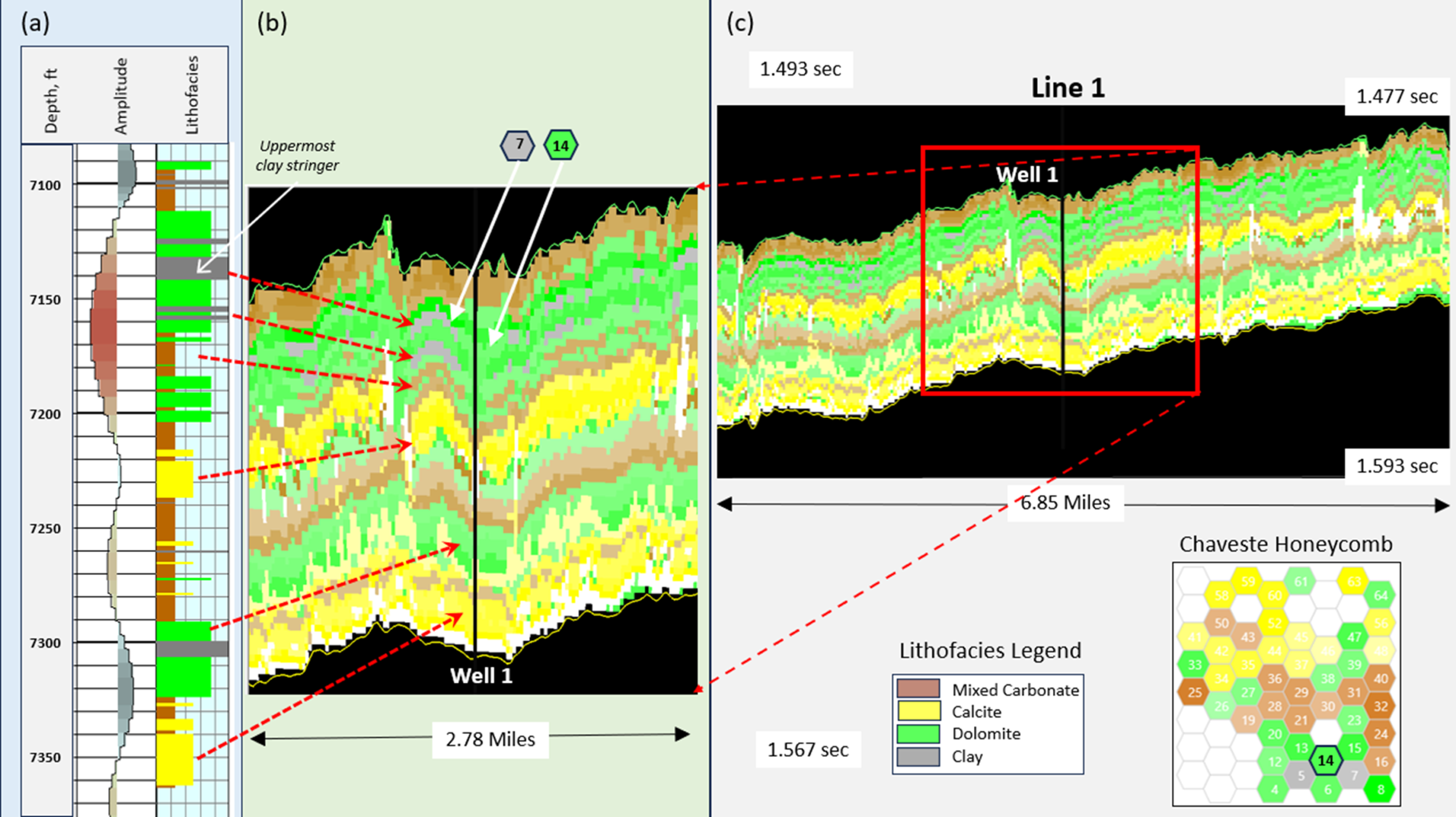
Paradise Lithofacies and Fault Prediction – Machine and Deep Learning Applications in the Permian Basin
Speaker: Robin Dommisse, Senior Geomodeling Advisor, Bureau of Economic Geology, University of Texas at Austin
3D reservoir characterization models of subsurface reservoirs rely on the maximum extraction of knowledge from the 3D seismic and well log data sets. In this presentation we discuss a new machine learning (ML) powered methodology for 3D lithofacies prediction of clinoform ooid grainstones in a Midland Basin carbonate CCUS reservoir. This new approach is faster than traditional seismic inversion techniques, leveraging the power of Self Organized Maps (SOM), an unsupervised form of machine learning. Using SOM, we create detailed 3D volumes of classified data down to a single seismic sample interval. These volumes are then combined with lithofacies from well logs, merging two independent data sources for more accurate predictions. In a second example we show how to quickly generate fault probability volumes for several 3D seismic surveys in the Permian Basin. The AI-based fault detection workflow discussed here uses a combination of supervised Deep Learning (DL) and unsupervised Machine Learning (ML) technologies to produce fault geobodies which are converted to fault planes for use in 3D sealed, structural geomodels.
Comparison to seismic inversion for lithofacies prediction
Paradise® delivers a breakthrough approach to lithofacies prediction—one that’s data-driven, faster, and more cost-effective than traditional seismic inversion. By applying advanced machine learning to seismic and well data, Paradise bypasses the constraints of deterministic models. There’s no need to define wavelets or low-frequency trends—saving time, reducing complexity, and eliminating key sources of uncertainty.
Paradise’s ML-based workflow analyzes multiple seismic attributes simultaneously, identifying natural patterns that correlate with lithology. The result is a high-resolution litho-stratigraphic model that classifies each seismic sample with the most probable lithofacies and associated probabilities. This model offers granular vertical and horizontal resolution, enabling interpreters to characterize both thin and thick beds with confidence—often below the limits of traditional inversion.
Unlike conventional methods, Paradise leverages Self-Organizing Maps (SOMs) to extract subsurface patterns from seismic data, then ties those clusters directly to facies from well logs. This powerful integration of independent data sources produces more geologically consistent models, with proven success across global basins.
Why it matters:
- No physical modeling or wavelet assumptions required
- Works at the seismic sample interval—below tuning thickness
- Reduces ambiguity through multi-attribute ML clustering
- Faster turnaround and lower costs than seismic inversion
- Aligns machine learning with interpreter judgment for better decision-making
Amplitude Trace and Seismic Attributes Computed from Amplitude
Multidimensional vectors input into SOM are obtained by taking the same time/depth sample for all attributes. Each sample is classified by a neuron, which provides interpretable data below the seismic wavelet resolution.
“SOMs find facies patterns first, which can then be labeled by the geologist using log data or geologic analogs. The flexible nature of SOMs makes them a useful unsupervised approach for seismic facies analysis, particularly where there may be limited well control.”
– Dr. Heather Bedle, assistant professor at the University of Oklahoma and principal investigator for the Attribute-Assisted Seismic Processing & Interpretation (AASPI) Consortium
SOM Topology with Seismic Amplitudes and Lithofacies
The SOM topology map at left corresponds to the SOM neurons in the middle track. The left-most well track shows the seismic trace at the well location. The right-most trak is the lithofacies computed from well logs. Formation thicknesses observed in the lithofacies are smaller than a seismic trough (reference the shallow clays).
Four Lithofacies Estimated from Petrophysical Evaluation of Five Niobrara Wells
The lithofacies from the well shown in the right-most track of Figure 2 is the well-log representation of the lithofacies shown here as PDF plots. The economic importance of the play is evidenced by the appearance or hydrocarbons in all lithofacies.
Lithofacies Prediction in the Niobrara Formation
The SOM-to-Lithofacies correlation ties the neurons from seismic data to the lithofacies from well logs. In the lower right, the ‘winning’ lithofacies topology shows the lithofacies corresponding to the neurons computed from seismic. The lithofacies from the well are inserted for reference at the well’s location. Multi-client data presented with permission from Geophysical Pursuit, Inc. & Fairfield Geotechnologies.
Supporting resources on lithofacies prediction using Paradise
Step 1: Discovery Call – Schedule Now
Schedule your introductory call to discuss what AI can do for you.
Start your AI implementation journey today with a short, 30-minute introductory call. We will listen carefully to your objectives and design a path for your team that realizes tangible benefits.
Step 1: Discovery Call – Schedule Now
Schedule your introductory call to discuss what AI can do for you.
Start your AI implementation journey today with a short, 30-minute introductory call. We will listen carefully to your objectives and design a path for your team that realizes tangible benefits.